- Twice the Impact PM π₯
- Posts
- The Right Way to Perform A/B Testing for Product Managers
The Right Way to Perform A/B Testing for Product Managers
Making Right Choices as Product Managers & Analysts β

Hi Impactful PM! Itβs Areesha :)
Imagine you're running a lemonade stand on a hot summer day. You want to see which sign attracts more thirsty customers, but you only have enough time and materials to make two versions.
Which one will you choose to beat the heat? π
Hereβs what you plan to do. You decide to create two versions of a sign to see for yourself what works for you.
Version A: A classic sign with a picture of a refreshing glass of lemonade and the words "Lemonade for Sale!"
Version B: The same picture, but with the words "Beat the Heat! Get Your Ice-Cold Lemonade Here!"
Here's how you can A/B test your signs:
Flip a coin: Heads - Version A goes on the left, Tails - Version B goes on the left. This randomizes which customers see which sign first.
Sell lemonade for an hour: Track how many people buy lemonade from each side of the stand.
Count the cups: Whichever side sold more lemonade has the winning sign!
This is a simple A/B test, but it helps you understand the core concept. Letβs take a deep dive into A/B testing in product management today!
Hottest from last week π₯
A/B Testing for Product Managers and Analysts
A/B testing is a cornerstone of data-driven product development. It allows product managers (PMs) and product analysts to compare different product features or element versions to see which performs better against a specific metric.
Benefits of A/B testing for Product Managers π
A/B testing offers a multitude of advantages for businesses, particularly for product managers and analysts.
Data-Driven Decisions: A/B testing replaces guesswork with concrete data. By comparing different versions of features or elements, you can see statistically what resonates more with your users. This data-driven approach ensures you're making informed choices for product development and optimization.
Improved User Experience (UX): A/B testing allows you to test various design elements and functionalities to see what users find most intuitive and engaging. This helps you refine your product for a smoother user experience, ultimately leading to higher satisfaction and retention.
Increased Conversion Rates: Whether your goal is getting users to sign up, subscribe, or make a purchase, A/B testing helps you identify what elements drive those desired actions. By optimizing your product based on winning test variations, you can significantly increase conversion rates.
Reduced Risk: A/B testing allows you to experiment with changes on a smaller scale before rolling them out to your entire user base. This mitigates the risk associated with implementing potentially disruptive changes, as you can identify what works and avoid pushing out features users might dislike.
Uncovering the Unexpected: A/B testing can sometimes reveal surprising results. You might discover elements that have a much bigger impact (positive or negative) than anticipated. This can lead to uncovering entirely new opportunities for product improvement you might not have considered before.
Faster Iteration: A/B testing allows you to continuously test and refine your product based on user data. This iterative approach ensures you're constantly optimizing for what works best, leading to faster product improvement cycles.
Prioritization: With A/B testing data, you can prioritize which features or changes to tackle first. By focusing on elements with the potential for the biggest impact based on test results, you can optimize your development resources and efforts.
Understanding the A/B Testing Process π€
The A/B Testing Process:
Define Your Hypothesis: This is the core of your test. What are you trying to learn? It could be anything from "A red button will increase click-through rates compared to a blue button" to "A simplified checkout process will lead to higher conversion rates."
Choose Your Metric(s): What will success look like? Common metrics include conversion rates, click-through rates, time spent on a page, or user engagement. Ensure your metric aligns with your hypothesis.
Design Your Variations: Create the two (or more for multivariate testing) versions you'll be testing. Be clear and concise about the differences between them. Focus on one variable per test for cleaner results.
Set Up Your Test: Use A/B testing tools or leverage your development team to implement the variations. Split your user base randomly into groups (usually 50/50) to ensure a fair comparison.
Run the Test: Let the test gather data for a statistically significant timeframe. This depends on your user traffic and the metric you're tracking. A/B testing tools often provide sample-size calculators to guide you.
Analyze the Results: Use statistical analysis to determine if there's a statistically significant difference between the variations. Tools will often provide this information alongside visualizations of the data.
Interpret and Act: Look beyond the p-value (statistical significance) and consider the magnitude of the effect. If the winning variation has a small positive impact, it might not be worth implementing. Use the results to inform future product decisions and potentially iterate on the winning variation.
Best Practices of A/B Testing β‘
Here are some best practices to ensure your A/B tests are effective and deliver actionable insights:
Planning and Design:
Start with a clear hypothesis: What are you trying to learn and how will the test help you achieve that? A well-defined hypothesis ensures your test is focused and delivers targeted results.
Focus on user needs: Align your tests with user research and pain points. Address what your users struggle with and how A/B testing can help solve those problems.
Prioritize impact: Test elements with the potential to significantly affect your key metrics (e.g., conversion rates, engagement). Don't waste resources on minor changes.
Test one variable at a time: Isolate a single variable per test (e.g., button color, layout) to pinpoint the cause of any observed effects and avoid muddying the results.
Execution and Analysis:
Sample size matters: Ensure your test gathers enough data to produce statistically significant results. Use a sample size calculator to determine the appropriate number of users for your test.
Run the test for long enough: Don't peek at results prematurely! Let the test gather data for a statistically significant timeframe, accounting for natural traffic fluctuations.
Analyze multiple metrics: Look beyond your primary metric and consider secondary metrics like bounce rate, time spent on a page, or user engagement to gain a holistic understanding of user behavior.
Segment your data: Analyze results by user groups (demographics, behavior) to see if variations resonate better with specific segments. This can reveal opportunities for targeted optimization.
Interpretation and Action:
Don't rely solely on p-value: Statistical significance is important, but consider the magnitude of the effect as well. A small improvement might not justify changes.
Consider external and internal factors: Did external events (marketing campaigns) or internal changes (product updates) occur during the test that might have influenced results? Account for these factors.
Document everything: Record your hypothesis, methodology, results, and learnings for future reference and to build upon your A/B testing knowledge.
Iterate and test again: A/B testing is a continuous process. Use learnings from past tests to refine hypotheses and design new experiments for ongoing product optimization.
Additional Best Practices:
Use A/B testing tools: Leverage A/B testing tools to streamline test setup, data collection, and analysis. These tools can also help ensure proper randomization and statistical rigor.
Communicate and collaborate: Keep stakeholders informed about A/B testing plans and results. Involve data analysts throughout the process to ensure data integrity and interpretation.
Test ethically: Ensure your tests comply with user privacy regulations and avoid manipulative practices that could negatively impact user experience.
Embrace the unexpected: A/B testing can sometimes reveal surprising results. Be open to learning from these unexpected outcomes β they can lead to new opportunities for product improvement.
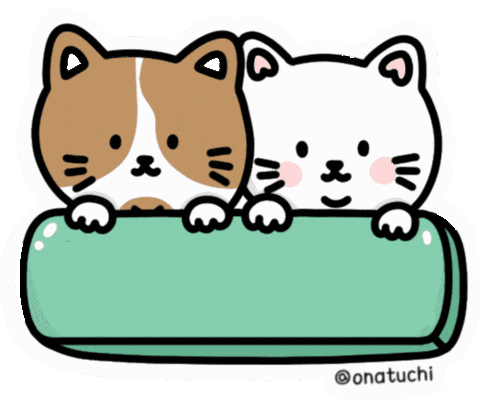
Gif by onatuchi on Giphy
Thatβs all for today !
π₯ How hot was this post? |
Stay tuned for some freshly baked PM tips, strategies, insights, weekly Q/A digests, and more right into your inbox!π
Cya!
Areesha β€οΈ
Connect with us on LinkedIn:
Reply