- Twice the Impact PM 🔥
- Posts
- Interview - What are the key challenges you anticipate when integrating AI into an existing product?
Interview - What are the key challenges you anticipate when integrating AI into an existing product?
The right way to answer this PM interview question

Hey Impactful PM! It’s Aneesha :)
As AI technologies continue to advance, many product managers face unique challenges in this integration process.
In this interview-style post, we’ll explore these challenges through a conversation that sheds light on what to anticipate when bringing AI into the mix. Let’s jump right in! 🚀
Looking for unbiased, fact-based news? Join 1440 today.
Upgrade your news intake with 1440! Dive into a daily newsletter trusted by millions for its comprehensive, 5-minute snapshot of the world's happenings. We navigate through over 100 sources to bring you fact-based news on politics, business, and culture—minus the bias and absolutely free.
Hottest from last week 🔥
Integrating AI Into an Existing Product
Understanding AI Integration Challenges
Interviewer:
To kick things off, what are the initial challenges you foresee when integrating AI into an existing product?
Interviewee:
Integrating AI into an existing product presents several initial challenges that we must be prepared for:
Data Quality and Availability: One of the foremost challenges is ensuring that we have high-quality, relevant data for training the AI models. In many cases, existing products may not have sufficient data, or the data may be fragmented or inconsistent.
Technical Compatibility: Another significant challenge is the technical compatibility of the existing product with new AI technologies. We need to assess whether our current infrastructure can support AI integration or if substantial upgrades are required.
Change Management: Introducing AI often necessitates changes in workflows, processes, and even team structures. Preparing the organization for these changes can be a daunting task, as it involves aligning various stakeholders and managing resistance.
These challenges highlight the complexity of AI integration and the importance of careful planning.
Observation
The interviewee provides a clear overview of the primary challenges faced during AI integration.
Their focus on data quality and availability indicates a strong understanding of the foundational role data plays in AI, while the mention of technical compatibility and change management reflects a comprehensive approach to addressing the multifaceted nature of integration challenges.
Addressing User Concerns
Interviewer:
That makes sense! How do user concerns factor into the integration of AI into existing products?
Interviewee:
User concerns are critical when integrating AI. Here are the main issues I consider:
Transparency: Users often want to understand how AI is making decisions, especially in sensitive areas like finance or healthcare. We must ensure that our AI systems are transparent and that users can trust the process.
Bias and Fairness: There’s also the risk of bias in AI algorithms, which can lead to unfair outcomes. We need to be vigilant in evaluating our models for bias and ensuring that they provide equitable results for all users.
User Experience: Finally, we have to ensure that the integration enhances the user experience rather than complicates it. If the AI features confuse or frustrate users, it could lead to negative perceptions of the product.
In summary, addressing user concerns around transparency, bias, and overall experience is essential for successful AI integration.
Observation
The interviewee’s emphasis on user concerns demonstrates a strong user-centric approach.
By prioritizing transparency and fairness, they show an awareness of ethical considerations in AI, while their focus on user experience highlights their commitment to maintaining product quality and user satisfaction.
Managing Integration Complexity
Interviewer:
Let’s talk about integration complexity. How do you foresee managing the complexity that comes with integrating AI into an existing product?
Interviewee:
Managing integration complexity is indeed a significant challenge. Here’s how I approach it:
Phased Implementation: I advocate for a phased implementation approach. By breaking down the integration into smaller, manageable phases, we can mitigate risks and gather feedback at each stage, making necessary adjustments.
Stakeholder Involvement: Involving stakeholders from the beginning can help identify potential challenges early on. Regular check-ins and updates will keep everyone aligned and engaged in the process.
Clear Documentation: Maintaining clear documentation throughout the integration process is essential. This helps ensure that everyone is on the same page and can refer back to the rationale behind decisions and processes.
By implementing these strategies, we can navigate the complexities of AI integration more effectively.
Observation
The interviewee offers practical strategies for managing integration complexity, demonstrating a strategic mindset.
Their emphasis on phased implementation and stakeholder involvement shows a commitment to risk management and collaboration, while clear documentation highlights their attention to maintaining organizational knowledge throughout the process.
🤣 Product Management Meme of the Day 🤣

đź’ˇ PM Productivity Tip of the Day đź’ˇ
Here are a few lines to help you keep going 🎉
Know the lay of the land: Understand your industry and context.
That’s all for today !
Stay tuned for some freshly baked PM tips, strategies, insights, weekly Q/A digests, and more right into your inbox!🚀
Cya!
Aneesha ❤️
Connect with us on LinkedIn:
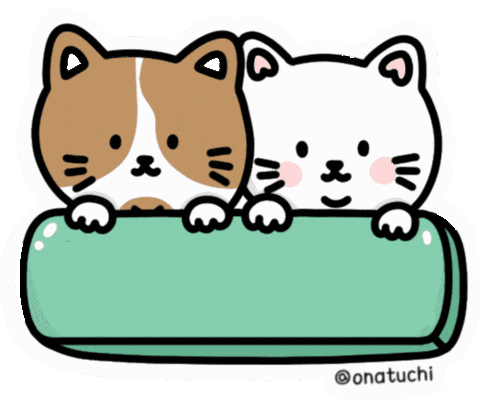
Gif by onatuchi on Giphy
Reply